- Who We Serve
- What We Do
- About Us
- Insights & Research
- Who We Serve
- What We Do
- About Us
- Insights & Research
Exploiting the Benefits of Artificial Intelligence for Factor Investors
Our research shows how artificial intelligence can potentially enhance performance of equity investing.
- Portfolio Construction
- Equity Insights
- Active Equity
- Factor Investing
Key Points
What it is
We demonstrate how artificial intelligence may enhance equity-factor performance through a method called dynamic factor timing.
Why it matters
Artificial intelligence can help address the problem that factors go through cycles with periods of underperformance.
Where it's going
Hypothetical tests show that dynamic factor timing may increase factor-based equity absolute and risk-adjusted returns.
Equity investors have long embraced — using factors such as , and — to improve portfolio performance. Driving factor utilization is research and empirical evidence supporting their persistent historical equity outperformance over the broad stock market over time.1 Meanwhile, investors have made a massive push to apply to their investment processes to boost performance and mitigate risk. Increased data availability and the advancement of artificial intelligence techniques — including the potential to identify and exploit new connections in financial markets — have contributed to the growing exploration in this exciting space.
One opportunity this raises resides at the intersection of these two ideas: factor investing and artificial intelligence. The area of the intersection we explore here is factor timing and more specifically, dynamic factor timing. To do so, we have partnered with University of Chicago Professor Stefan Nagel, one of the world’s leading experts on factor investing and artificial intelligence.2 Together, we explore how investors can apply factor investing and artificial intelligence in the context of traditional modern portfolio theory.
Artificial Intelligence: Avoid the Pitfalls to Potentially Enhance Outcomes
Artificial intelligence can potentially improve investment outcomes because of its ability to process large amounts of data in order to uncover insights. This is more specifically called , a subfield of artificial intelligence. Yet investors are just getting started on how to use it. The exciting possibilities include better predictions of return and risk, more efficient portfolios and the uncovering of hidden patterns. However, these techniques also come with significant user warnings. Common pitfalls that can undermine their effectiveness include misapplication, overreliance on single and non-transparent estimates, and over-fitting models to historical data.
To capitalize on the benefits while avoiding the pitfalls in a factor timing context, our research shows that investors can apply a robust investment process driven by artificial intelligence. This approach shows how we can improve factor timing techniques through a more dynamic, as opposed to static, approach.
Factor Timing: From Static…
Factors go through cycles over which their returns vary. One step investors can take to mitigate this cyclicality is to diversify across factors. An important question that emerges is whether investors can time this cyclicality. Briefly, factor timing involves identifying relationships between variables such as sentiment, valuation, or the current business cycle and how they impact factor returns. For example, research may reveal that factors have historically performed well will continue to do so. In a timing framework, this means we should overweight factors that have shown recent positive performance, also known as momentum, and vice versa.
Most prevailing factor timing techniques are static in nature. While the relative preference of factor weightings does change, a static approach ignores the dynamic relationship between predictor variable and factor over time. For example, perhaps using momentum to time factors works overall, but there are times when it does not.
…To Dynamic
Recognizing that, in reality, factor relationships are not constant, we aimed in our research to build upon static approaches and create a dynamic approach to capture changing relationships in a factor timing approach. To do so, we developed a dynamic factor timing framework that sits at the intersection of modern portfolio theory and artificial intelligence.3
Specifically, we applied a standard approach — the cornerstone of modern portfolio theory — along with an artificial intelligence technique known as regularization. Regularization is an approach that, for our purposes, applies data-driven skepticism to the expected success of factor timing. This process digests incoming data in a systematic manner to dynamically adjust the factor weights to produce the optimal allocations for a dynamic factor timing portfolio.
Should factor timing be deemed temporarily ineffective, the regularization approach could dial down — or even turn off — factor timing and revert to an equal weighting of the targeted factors in a portfolio (equal-weighted factor portfolio). This can happen when the degree of skepticism is high, as determined by artificial intelligence. Alternatively, when the model is confident and the degree of skepticism is low, the portfolio tilts toward the dynamic factor-timing portfolio, where artificial intelligence will dial up the emphasis on the factor timing portfolio.
The Results
Using this framework, we evaluate results from a long-only portfolio comprising the value, momentum and quality4 factors. Exhibit 1show the benefits to return and risk from our dynamic factor timing portfolio. We first note that the equal-weighted factor portfolio provided improved results versus the excluding the financial sector, which is consistent with the literature on factor investing.
Second, we see the further return improvement of the dynamic factor portfolio. Specifically, this portfolio, which incorporates the static factor timing approach along with the artificial-intelligence implementation its importance, further improved performance by 1.5% a year on average with somewhat lower risk. Combining these, we can see a meaningful pick-up in Sharpe ratio from 0.66 for the index to 0.75 for the equal-weighted factor portfolio all the way up to 0.82 for the dynamic factor portfolio. Overall, the results of this methodology demonstrate substantial benefits for return and risk in a multi-factor portfolio.
Factor-based strategies provide exposure to stocks with similar pre-defined characteristics such as quality, low-volatility, value, high-dividend, small-size and momentum. These factors have historically outperformed the broad market over time.
This factor targets companies with efficient management, profitability, and strong cash flows. Quality strategies seek to provide excess returns by investing in companies that are better positioned for short and long term growth.
The Russell 1000 Index measures the performance of the large-cap segment of the U.S. equity universe.
Mean-variance analysis is an approach to portfolio construction using expected means, variances and covariances of asset returns. Variance is a measure of dispersion of a variable about its mean. Covariance is a measure of co-movement between two variables.
The momentum factor targets companies that have strong market sentiment and analyst sentiment.
Artificial intelligence is the capability of computer systems or algorithms to imitate intelligent human behavior.
Machine learning is an artificial intelligence process in which computer systems identify patterns in datasets to make or refine the decisions and predictions that they generate without being explicitly programmed to do so.
The value factor targets companies that trade at a low price relative to a fundamental measure.
Exhibit 1: Hypothetical: The Outperformance of Dynamic Factor Timing
Our hypothetical dynamic factor portfolio outperformed the broad market, excluding the financial sector, and an equal-weighted factor portfolio on an absolute and risk-adjusted return basis.
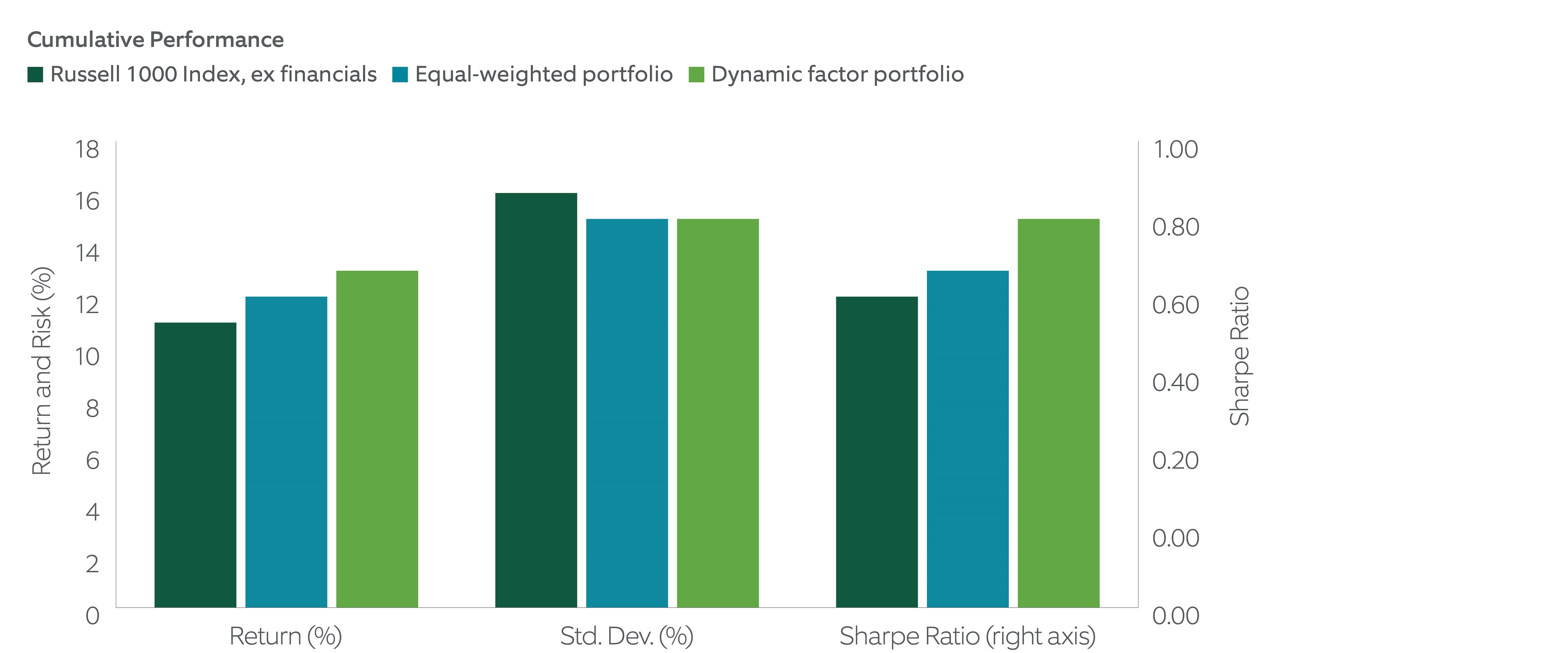
Sources: Northern Trust, FTSE-Russell, FactSet, CRSP, FRED. Data from December 31, 2005 to December 31, 2023. For illustrative purposes only. Actual results may vary. Research based on the working paper Optimal Factor Timing in a High-Dimensional Setting, by Lehnherr, Mehta and Nagel, September 2024. This graph does not show actual performance results. Index performance returns do not reflect any management fees, transaction costs or expenses. It is not possible to invest directly in any index.
Enhancing Traditional Portfolio Management with AI
When it comes to both artificial intelligence and timing anything in financial markets, it is crucial to apply discipline. Merely identifying and exploiting relationships that are economically meaningful and showing positive long-run average results can ignore how those relationships change over time. Traditional methods may not pick up these changes, while artificial intelligence approaches are well-suited to identify them and adjust. Yet we do not want to deviate too far from the proven practice of more traditional .
Therefore, leveraging our partnership with Professor Nagel, we have developed a flexible framework at the intersection of traditional portfolio theory and artificial intelligence that addresses these issues. This framework enhances the construction of factor timing portfolios by allowing for adjustments to market environments that can perniciously impact static factor timing. Using a dynamic framework, we adjust the degree of timing, and can even shut off all timing, should our approach pick up on a hidden change in predictor variable-factor relationship. We anticipate several important implications of this methodology, providing investors with enhanced tools for navigating increasingly complex financial markets.
1 See, for instance, Hunstad, Lehnherr, Prakash (2023) Foundations in Factors, Northern Trust White Paper.
2 Professor Nagel is former editor of the Journal of Finance and author of a key AI/ML textbook. This collaboration has yielded a unique artificial intelligence-based framework — a framework that recognizes many pitfalls of artificial intelligence techniques — and applied it to timing of factor returns and risks.
3 For full technical details, see Robert Lehnherr , Manan Mehta, and Stefan Nagel paper Optimal Factor Timing in a High-Dimensional Setting, available at SSRN 4938729 (2024).
4 Variables used in this analysis each factor were: cash flow yield, 12-minus-1, total asset growth, and gross profitability, respectively.
Main Point
A potentially more dynamic approach to factor investing with AI
Factor relationships are not constant, so we aimed in our research to build upon static approaches and create a dynamic approach to capture changing relationships in a factor timing approach. We believe this framework enhances the construction of factor timing portfolios by allowing for adjustments to market environments that can negatively impact static factor timing.
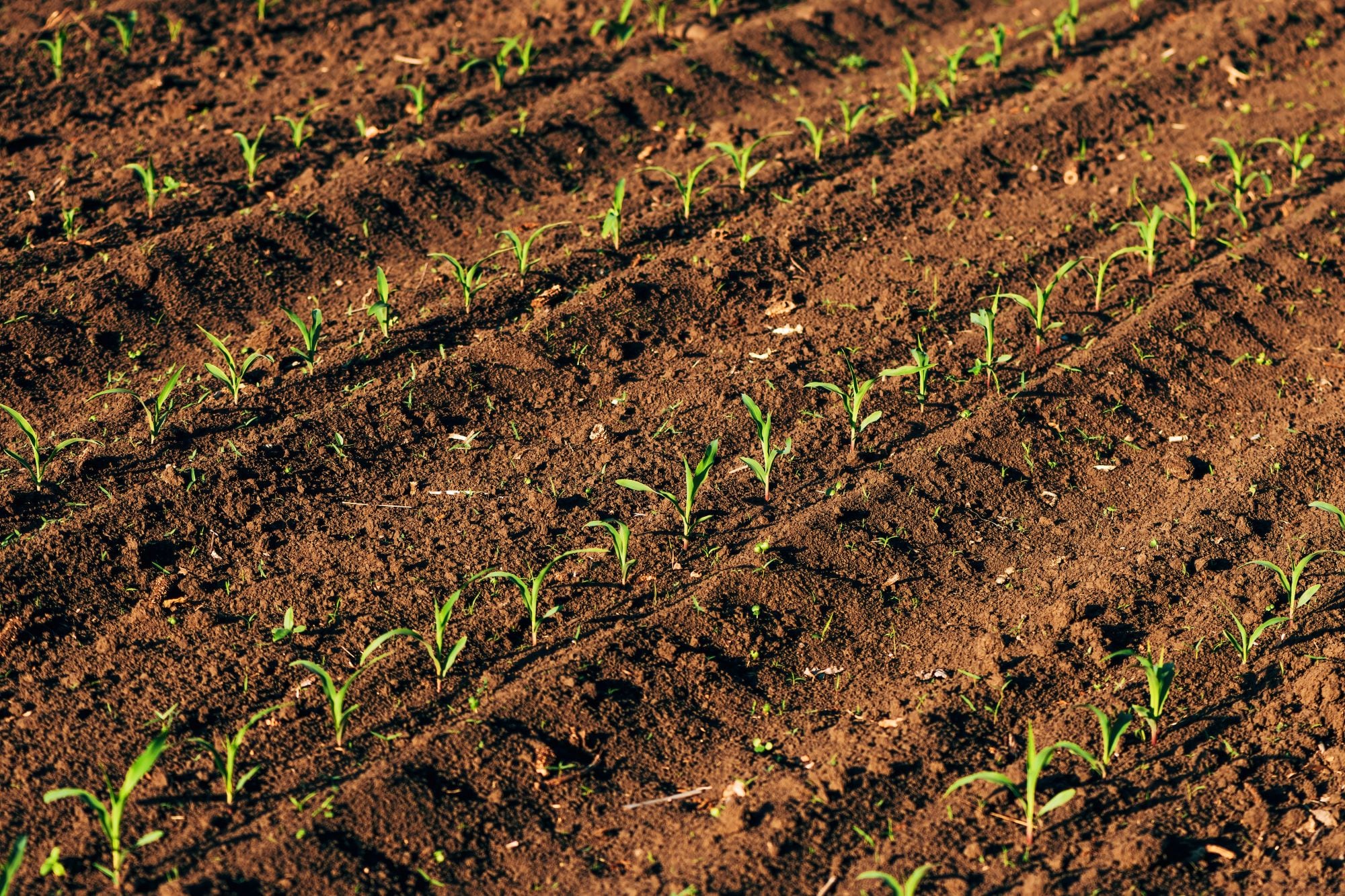
Contact Us
Interested in learning more about our expertise and how we can help?
IMPORTANT INFORMATION
For Asia-Pacific (APAC) and Europe, Middle East and Africa (EMEA) markets, this information is directed to institutional, professional and wholesale clients or investors only and should not be relied upon by retail clients or investors. The information contained herein is intended for use with current or prospective clients of Northern Trust Investments, Inc (NTI) or its affiliates. The information is not intended for distribution or use by any person in any jurisdiction where such distribution would be contrary to local law or regulation. NTI or its affiliates may have positions in and may effect transactions in the markets, contracts and related investments different than described in this information. This information is obtained from sources believed to be reliable, its accuracy and completeness are not guaranteed, and is subject to change. Information does not constitute a recommendation of any investment strategy, is not intended as investment advice and does not take into account all the circumstances of each investor.
This report is provided for informational purposes only and is not intended to be, and should not be construed as, an offer, solicitation or recommendation with respect to any transaction and should not be treated as legal advice, investment advice or tax advice. Recipients should not rely upon this information as a substitute for obtaining specific legal or tax advice from their own professional legal or tax advisors. References to specific securities and their issuers are for illustrative purposes only and are not intended and should not be interpreted as recommendations to purchase or sell such securities. Indices and trademarks are the property of their respective owners. Information is subject to change based on market or other conditions.
All securities investing and trading activities risk the loss of capital. Each portfolio is subject to substantial risks including market risks, strategy risks, advisor risk, and risks with respect to its investment in other structures. There can be no assurance that any portfolio investment objectives will be achieved, or that any investment will achieve profits or avoid incurring substantial losses. No investment strategy or risk management technique can guarantee returns or eliminate risk in any market environment. Risk controls and models do not promise any level of performance or guarantee against loss of principal. Any discussion of risk management is intended to describe NTI or its affiliates’ efforts to monitor and manage risk but does not imply low risk.
Past performance is not a guarantee of future results. Performance returns and the principal value of an investment will fluctuate. Performance returns contained herein are subject to revision by NTI or its affiliates. Comparative indices shown are provided as an indication of the performance of a particular segment of the capital markets and/or alternative strategies in general. Index performance returns do not reflect any management fees, transaction costs or expenses. It is not possible to invest directly in any index. Net performance returns are reduced by investment management fees and other expenses relating to the management of the account. Gross performance returns contained herein include reinvestment of dividends and other earnings, transaction costs, and all fees and expenses other than investment management fees, unless indicated otherwise. For additional information on fees, please refer to Part 2a of the Form ADV or consult an NTI representative.
Forward-looking statements and assumptions are NTI or its affiliates’ current estimates or expectations of future events or future results based upon proprietary research and should not be construed as an estimate or promise of results that a portfolio may achieve. Actual results could differ materially from the results indicated by this information.
This information is intended for purposes of NTI and/or its affiliates marketing as providers of the products and services described herein and not to provide any fiduciary investment advice within the meaning of Section 3(21) of the Employee Retirement Income Security Act of 1974, as amended (ERISA). NTI and/or its affiliates are not undertaking to provide impartial investment advice or give advice in a fiduciary capacity to the recipient of these materials, which are for marketing purposes and are not intended to serve as a primary basis for investment decisions. NTI and its affiliates receive fees and other compensation in connection with the products and services described herein as well as for custody, fund administration, transfer agent, investment operations outsourcing, and other services rendered to various proprietary and third-party investment products and firms that may be the subject of or become associated with the services described herein.
Northern Trust Asset Management is composed of Northern Trust Investments, Inc., Northern Trust Global Investments Limited, Northern Trust Fund Managers (Ireland) Limited, Northern Trust Global Investments Japan, K.K., NT Global Advisors, Inc., 50 South Capital Advisors, LLC, Northern Trust Asset Management Australia Pty Ltd, and investment personnel of The Northern Trust Company of Hong Kong Limited and The Northern Trust Company.
Not FDIC insured | May lose value | No bank guarantee